Congratulations to our team's PhD student Jianing He on the acceptance of the paper titled “Improving Prediction Certainty Estimation for Reliable Early Exiting via Null Space Projection” at IJCAI 2025
A paper titled "Improving Prediction Certainty Estimation for Reliable Early Exiting via Null Space Projection" by Jianing He, a PhD student from our team, has been accepted by IJCAI 2025. IJCAI (International Joint Conference on Artificial Intelligence) is one of the premier academic conferences in the field of artificial intelligence. As an A-level conference recommended by the China Computer Federation, IJCAI plays a crucial role in promoting academic exchange and collaboration, providing researchers with the opportunity to engage with the latest advancements in AI. This paper addresses the issue of prematurely exiting samples with incorrect predictions in traditional logit-based early exiting methods, which arise from neglecting class-irrelevant information in the features. To achieve this, we propose a novel early exiting method based on the Certainty-Aware Probability (CAP) score, which integrates insights from both logits and the NSP score to enhance prediction certainty estimation and facilitate more reliable exiting decisions. Experimental results on the GLUE benchmark demonstrate that our method improves inference speed by 28% over the strong baseline ConsistentEE, while maintaining comparable task performance, thereby providing a better trade-off between performance and efficiency in pre-trained language models.
IJCAI(国际人工智能联合会议)是人工智能领域的顶级学术会议之一。作为中国计算机联合会(CCF)推荐的A类会议,IJCAI在促进学术交流与合作方面发挥着至关重要的作用,是研究人员进行交流和了解人工智能前沿动态的重要平台。
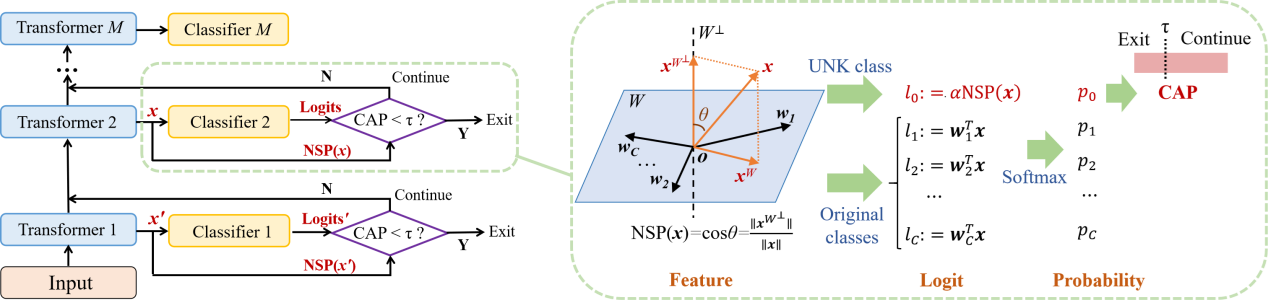
提前退出方法通过在推理阶段动态调整每个样本的执行层数,有效提升了预训练语言模型的推理效率。然而,现有的提前退出方法主要依赖类别相关的logit来构造退出信号,以估计预测确定性,忽视了特征中的类别无关信息对预测确定性的负面影响。这导致了对预测确定性的过高估计,使得模型倾向于过早地退出那些预测结果尚且错误的样本。为解决这一问题,本文设计了一种零空间投影(Null Space Projection, NSP)分数,通过考虑特征中类别无关信息的比例来估计预测确定性。在此基础上,本文进一步提出了一种基于确定性感知概率(Certainty-Aware Probability, CAP)分数的提前退出方法,该方法将类别相关的logit和类别无关的NSP分数进行整合,以增强预测确定性估计,从而实现更可靠的退出决策。基于GLUE基准的实验结果表明,与强基线方法ConsistentEE相比,提出的方法在保证相同任务性能的前提下,推理速度提高了28%,更好地平衡了预训练语言模型的任务性能和推理效率。