Congratulations to our team's PhD student Jianing He on the acceptance of the paper titled “COSEE: Consistency-Oriented Signal-based Early Exiting based on Calibrated Sample Weighting Mechanism” at AAAI 2025
A paper titled "COSEE: Consistency-Oriented Signal-based Early Exiting based on Calibrated Sample Weighting Mechanism" by Jianing He, a PhD student from our team, has been accepted by AAAI 2025. AAAI conference is organized by the Association for the Advancement of Artificial Intelligence, is one of the top-tier academic conferences in the field of artificial intelligence. As a A-level conference recommended by the China Computer Federation, AAAI is an essential venue for academic exchange, allowing researchers to stay at the forefront of AI developments. This paper aims to address the inconsistency between training and testing in early exiting networks while ensuring flexible adjustments to the speed-up ratio. To achieve this, we propose a novel consistency-oriented training framework for signal-based early exiting networks, called COSEE. This framework leverages a calibrated sample weighting mechanism to enable each classifier to emphasize samples that are more likely to exit at that classifier under various acceleration scenarios. Extensive experiments demonstrate the effectiveness of the COSEE design.
AAAI是人工智能领域的顶级学术会议之一,由美国人工智能协会(AAAI)主办。会议每年举行一次,旨在展示和交流人工智能各个领域的最新研究成果、技术进展和应用实践。会议的内容涵盖了人工智能的多个方面,包括机器学习、自然语言处理、计算机视觉、自动推理、智能系统、机器人学等。作为中国计算机学会(CCF)推荐的A类会议,AAAI是研究者进行学术交流和了解人工智能前沿动态的重要平台。
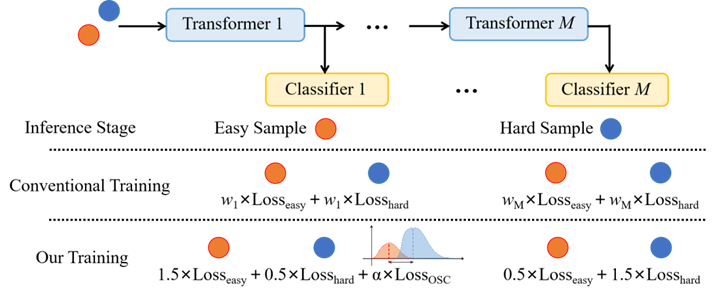
提前退出方法通过动态调整每个样本的执行层数,有效提升了预训练语言模型的推理效率。然而,现有研究在训练过程中通常无差别地对待所有样本,忽视了测试阶段样本的动态退出行为,导致训练与测试之间的不一致性。为此,本文提出了 COSEE,一种一致性导向的提前退出网络训练框架。该框架根据模拟的样本退出层数,为每个样本在所有分类器上的损失分配权重,确保样本在训练过程中能够被其潜在退出层附近的分类器所关注,从而缩小训练与测试之间的差距。此外,本文还引入了在线信号校准目标,以提高退出信号对样本难度的判别能力,从而确保损失权重分配的合理性,并增强推理阶段退出决策的可靠性。大量实验验证了 COSEE 设计的有效性,证明其不仅提升了提前退出网络的训练效果,还保证了灵活的模型加速比调节。